Today's episode is the second time that friend and podcast guest Jamie Flinchbaugh has turned the tables by playing host to interview me about my new book, Measures of Success: React Less, Lead Better, Improve More, which has recently been the #1 best selling book in Amazon's Total Quality Management category (yes, that's still a category, even though it seems like an outdated term for books about Lean, Six Sigma, etc.)
Jamie is very knowledgeable on these topics, so he was a great person to interview me and to have more of a conversation about choosing the right metrics and then managing them the right way. I hope you enjoy the conversation and future podcasts will return to the usual format where I interview guests and try to let them do most of the talking.
Streaming Player:
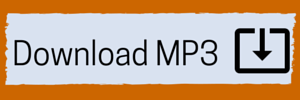
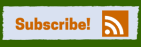
For a link to this episode, refer people to www.leanblog.org/316.
For earlier episodes of my podcast, visit the main Podcast page, which includes information on how to subscribe via RSS, through Android apps, or via Apple Podcasts. You can also subscribe and listen via Stitcher.
Questions, Topics, Links:
- Jamie's website | Twitter @flinchbaugh
- Mark's book
- What inspired you to write this particular book?
- Could you help us understand where does variation fit in into this universe of lean thinking?
- Dp you see this more as a management book or is there just as much in there for the individual contributors in an organization?
- If someone doesn't have that background, if this is new to them, is this going to be hard? How do we make it easier for someone that doesn't have that basic understanding of how this works?
- What's your advice on not overreacting to every data point?
- A lot of this comes down to problem statements, where a shift-the-mean problem statement is different than a variation reduction problem statement is different than a trend problem statement. I guess it's not about not reacting. It's about how to react.
- Let's talk a little bit about metric design
- People are either going to react or they're not. That's fundamentally outside my control. How does that influence the behaviors that you're seeking through the book?
- Will it kill the simplicity of the process chart because we have AI to figure these things out for us?
What are your hopes about the influence? Where does it go from here? What do you hope to change because of this book?
Check out the previous “audiobook” podcast from the book:
Full, Annotated Transcript:
Jamie Flinchbaugh: Hello, everyone. Thank you for listening to the podcast. We have a guest host, which is me, Jamie Flinchbaugh. I am your guest host. The reason for that is that our guest today is the regular host, Mark Graban. He's here to talk about his new book that's just out.
Mark, I want to just talk about the idea of the book. You've been really known for writing about lean in healthcare for several books and certainly a lot of the content on the blog. What inspired you to write this particular book?
Mark Graban: Thanks, Jamie. Thank you for playing guest host again here as we did once, I think just once before.
Note: My hair was a bit darker 10 years ago…
Mark: Yeah. The book was something a long time in the works. Just give a little bit of the background, I started my career, a lot of listeners might know, in the auto industry. When I was at General Motors in 1995 on the shop floor at GM, they had control charts, statistical-process-control charts.
These are methods that date back well into the early 20th century. Statistical control, SPC, charts have traditionally been used to measure and help manage quality of manufactured processes. Then GM was starting with a lean journey. My career has really been focused on lean.
With my roots in industrial engineering, I got exposed to SPC in college. In that time when I was working at General Motors, I was fortunate that my dad, who worked as an engineer at GM for 40 years before retiring, had a couple of books on his shelf that had piqued my interest.
One was the book “Out of the Crisis” by W. Edwards Deming. The second book was called “Understanding Variation — The Key to Managing Chaos” by Donald Wheeler. Wheeler was a student of Deming's. Deming was an advocate for statistical process control, learning how to understand and manage variation, which is the theme that Dr. Wheeler teaches so well in his books.
I was fortunate to be exposed to some of these concepts early in my career that are very, very helpful for managing a process, managing improvement. Throughout my career, I've always noticed that these methods seem to generally not be part of lean management practices, even though Toyota uses SPC charts. It seems like there's this gap or hole in practice, especially in healthcare.
...these methods seem to generally not be part of lean management practices, even though Toyota uses SPC charts. #MeasuresOfSuccess Share on XSeeing some of the dysfunctions that come from the way people generally track and manage metrics over time, I've been trying to teach these concepts in different formats in healthcare with workshops. I've been teaching a workshop a lot the last two years. I thought a book would be another helpful way to try to get some of these concepts in front of people.
Jamie: The idea of where this fits, the fact that you see many people in the lean world not appreciating the role of SPC charts, I remember back in the beginnings of our Chrysler Operating System 25 years ago that it was one of our four fundamentals that we listed.
Variation as a topic doesn't get as much attention in the lean community as the word waste. Could you help us understand where does variation fit in into this universe of lean thinking?
Mark: I agree with you on that. We can frame variation in terms of variation in the work and the variation in results. I think you agree with me, here. Some listeners might not agree but I'll say it anyway. The lean Six Sigma construct that often gets thrown around is you'll hear people say, “Lean is about reducing waste. Six Sigma is about reducing variation.”
Six Sigma does focus a lot on reducing variation. Some people might consider statistical process control to be a Six Sigma tool, even though, again, it predates even the TQM days when SPC was a big part of TQM.
Lean, if you look and say, “I think we realize the right process brings the right results,” even in my background, which is almost completely lean as opposed to Six Sigma. I've been taught that standardized work is one way we try to reduce variation in how the work is done to then reduce variation in our results.
That's one aspect of lean that contributes to reducing variation, and more importantly, improving results. If we look at variation and metrics, this is a big theme of my book, “Measures of Success.” If we're looking at a chart, whether it's on the shop floor, in a nursing unit, in the executive suite one lesson that Dr. Wheeler teaches so clearly is that there is variation in every metric.
The question is, how much variation is typical or routine? SPC charts, or in my book I've adopted Wheeler's terms, “process behavior charts.” A process behavior chart helps us understand from a baseline of data, here's the range in which our metric is varying or fluctuating. The same process, even if it's a very, very consistent process, won't always generate identical results.
There's variation in results due to many, many different factors in our system. Process behavior charts help us filter out all of the noise in that metric so that we're not reacting to every single up and down in a chart. We can use the basic math and principles of process behavior charts to see when there's been a meaningful change in our system.
Going to the subtitle of the book, “react less, lead better, improve more.” If we react less, we don't react equally to every up and down in the chart. That allows us to focus our attention. It allows us to focus our improvement efforts, which allows us to improve more. There's a different leadership style here that's not just knee-jerk reactive.
It's easy, anybody can say, “That number's worse than the target. That number's worse than last week. React. Explain. Give me a root cause.” There is no root cause for that routine variation. There is no root cause for noise in a system.
It's easy, anybody can say, That number's worse than the target. That number's worse than last week. React. Explain.#MeasuresOfSuccess Share on XThe rules of process behavior charts that help us detect signals, that's a statistically valid signal that says something has changed, whether that's good or bad, whether we're confirming the effect of an intentional change that we made or we're discovering, “Oh, wow. Something changed. We better figure out what that is.” Those are some of the ways that these charts and this methodology can be helpful.
There is no root cause for that routine variation. There is no root cause for noise in a system. #MeasuresOfSuccess Share on XJamie: That's great. That's a pretty good summary of the heart of the book. With that explanation in mind, do you see this more as a management book or is there just as much in there for the individual contributors in an organization?
The rules of process behavior charts that help us detect signals, that's a statistically valid signal that says something has changed, whether that's good or bad. #MeasuresOfSuccess Share on XMark: I definitely tried to write it as a management book that gives some simple statistical methods that help us manage better as opposed to a statistical…It's not a statistics textbook. I'm hoping the book is helpful to leaders at different levels, executives looking at their charts on their strategy deployment wall.
It can be helpful for middle managers, front-line managers who have their metrics that they're tracking at the front line, and to help better focus and connect their improvement work to the results, looking at cause and effect relationships between what we're doing differently or what we're not doing differently, and the impact on our results.
There's also an audience there in terms of the continuous improvement specialists, the lean people, the Kaizen people, the Six Sigma people. I talk about it in the book.
There are a lot of cases where there are some faulty statistical analysis that looks at one data point and says, “Oh, that data point's higher. Therefore, our project was a success. We can prove that we've improved,” when they might be declaring victory too soon. It might be a data point that falls into that range of noise or routine variation.
There are a lot of cases where there are some faulty statistical analysis that looks at one data point and says, oh, that data point's higher. Therefore, our project was a success. Share on XA lot of it's unintentional. Nobody's trying to lie with statistics but I think there are some methods that people haven't been exposed to that would be more valid ways of proving that we've made a significant and sustained shift in our metric.
Coming at it from one other direction, when I've been teaching workshops on these methods the last two years I've had a couple Six Sigma master black belts take my class, which that's a little intimidating. They probably know a lot of the deep, hardcore statistics better than I do.
The process behavior charts are considered, Wheeler uses the phrase, “It's the Swiss Army Knife of different types of control charts.” It's really robust. It can be used for all sorts of different metrics. It works really well in real-world circumstances, whereas a Six Sigma master black belt might have eight different types of variations of control chart in their arsenal.
The master black belts have all said they've appreciated that the methods that I share in my book here are less confusing to leaders. We're not nitpicking about all these different types of control charts. We have a method that helps us understand the variation in our process to help us prove if we've improved.
This is, from a practitioner's standpoint, really effective stuff. I've been happy to get that feedback, that master black belts could go back. What matters is what's effective. What are people willing to adopt in an organization? These methods are really useful and really practical.
Jamie: That's great. Freeing the management challenges in this, the book, it's not about a Statistics 101 book. There's lots of other places you can learn that. For myself, I feel pretty blessed. I took statistics in high school, at Lehigh, and Michigan, MIT, again at Chrysler. I got a lot of that over the years.
I have a base root skill that I understand how this stuff works. If someone doesn't have that background, if this is new to them, is this going to be hard? How do we make it easier for someone that doesn't have that basic understanding of how this works?
Mark: That's a good question. In the book, I focus a lot on the methods for interpreting process behavior charts. How do we identify signals? How do we identify shifts in our metric? It comes down to three rules that we can keep in our mind as we're looking at a chart. Those rules are, and in a podcast format like this it's hard to, what I'm trying to describe, what are very visual rules.
We're looking at a chart. We calculate an average. We calculate what are called lower and upper limits that tell us, “Here's the range in which we expect this process, this metric, to fluctuate over time.”
If we see any data point outside of those limits, this methodology tells the user of the chart, “That's unlikely to be randomly occurring. It's very, very, very likely, 99 percent likely, that there's been some change to the system.” That rule is easy to see on a chart. That is an opportunity then to go, react and do some root cause analysis.
The second rule is looking for eight consecutive data points that are on the same side as our average. Typically, a process is fluctuating. The metric is fluctuating around an average. It might not be exactly 50 percent above average and 50 percent below but it's fluctuating.
The second rule, it's very statistically unlikely that that would be randomly occurring. If we see those eight data points on the same side of the average that suggests that the process and our results have shifted either in a good direction or a bad direction.
There's a third rule that looks for a clustering of data points that are closer to the upper or lower limits in that range. Those three rules, as a user or consumer of process behavior charts, I think are pretty straightforward. The math for calculating an average, the math for calculating a lower and upper limit, is arithmetic. It's not calculus. There's not Greek letters involved, necessarily.
It's something that can be done in a pretty basic spreadsheet. It could be done by hand with paper and pencil, if need be, use the calculator on your iPhone. That's part of the beauty of the methodology. The biggest barrier, again, is that people haven't been introduced to these concepts. When people do get introduced to it they say, “OK, that's not that hard.”
Now we have to change management challenges of, how do you get leaders or an organization to step back and say, “The way we've been tracking metrics on a computer screen, or on a bulletin board, has maybe not been state of the art statistical method.” A lot of times people stay latched onto, “This is how I was taught to do it.”
Like you said, Jamie, I was fortunate that I was taught to do it this certain way. I got exposed to these ideas from Deming and Wheeler very, very early in my career. I had far less to unlearn. It's a huge challenge to try to get somebody to look back and say, “This is how I've been doing metrics for 20 years. This is how I was taught. That must be good.” That's challenging, right?
Jamie: Absolutely. There's two sides of the coin here. One is the, how to property react once you have the metrics in place to drive an understanding of what's going on with variation. The other is when not to react or overreact. That part almost seems harder than the how to react part.
Whether your kid brings home a bad report card, you have a quarterly miss on your financials, or you have a really upset customer you have these one offs that feel like you're compelled to act. What you're really saying is, “Don't necessarily react to single data points.” That sounds very difficult.
What's your advice on that side of the coin?
Mark: This is something where, over the last five years when I started making more effort to try to teach these methods in different formats. I've given some short talks at some conferences.
The way I was explaining the idea of, “Don't overreact to any single data point,” was leaving the impression, I could tell from the questions I was getting at the end about, “So you're saying if it's just noise, then there's nothing we can do about it?” I realized I was accidentally leaving that impression. I've tried to frame it differently.
If we're not reacting to a single data point, we're not wasting time asking for a root cause for a relatively small change in a metric. If we're looking at our average performance for a metric, if we're looking at the range of our lower and upper limits that are calculated from the voice of the process, we might have a process — I see this a lot — where people set a goal that's suspiciously close to last year's average performance.
If we're not reacting to a single data point, we're not wasting time asking for a root cause for a relatively small change in a metric. #MeasuresOfSuccess Share on XThe old rule of thumb might have been, “You need to react every time the metric is worse than the target.” Now you draw a process behavior chart. You see the metric's fluctuating around the average. It's a predictable process that's likely to continue fluctuating around that average and within those limits.
I'd look and say, “Well, if this process is not meeting our target, our customer need, however that target is set, half the time, we certainly need to improve the process or improve the underlying system.” This is where I think in the book I try to connect to less reactive, more systematic methods of improvement.
Listeners here would recognize A3 problem solving. They might recognize the language that Toyota uses of having a gap. We can close that gap by understanding our current state, our current process. We're going to propose some changes to that system that we think will boost the average performance of that system.
There's terminology that has been made more popular in lean healthcare circles when we talk in the context of lean management systems, driver metrics and watch metrics. Do you hear that terminology in other settings, Jamie?
Jamie: Not a lot, but I think it's pretty self-explanatory, those terms, though.
Mark: A driver is a metric where it's not meeting the target. You need to drive improvement through A3s. A watch metric is something that is meeting performance goals. We're just watching to see if it degrades. Where I think people get tripped up is with a driver, they might be reacting to every single data point that fluctuates just a little bit below the target.
Then they demand, “You need to find a root cause.” That root cause might not be there. They end up wasting a lot of time. The metric fluctuates back into the green range for no reason other than it just fluctuates.
Using the three rules of process behavior charts are a better indication of when something has changed. If we have a metric that's not consistently in that good performance range, we're still going to drive systematic improvement instead of reactive improvement.
The other thing I would say about the watch metric is the best situation — this is easier to visualize than to say verbally — if we have a metric where up is good and the lower limit of our process behavior chart is better than the target, I can feel really confident that that predictable system is going to continue to always exceed our target.
Where process behavior charts help is that we can look for these signals that the system is degrading and react before performance dips below the target. The way I see a lot of people articulating rules of thumb is, watch the metric. Watch the metric until it's no longer better than the target.
That might be kind of a late, slow reaction compared to using the process behavior rules that tell us, “Well, the system is degraded. It's still better than the target, but we need to react so we can put things back to where they were.” Is that…?
[crosstalk]
Jamie: Yeah, a lot of this comes down to problem statements, where a shift-the-mean problem statement is different than a variation reduction problem statement is different than a trend problem statement. I guess it's not about not reacting. It's about how to react.
Mark: I think knowing when to ask for a root cause for that day, that week, that month, as opposed to stepping back and saying we need to improve the underlying system, those are different reactions.
Jamie: Absolutely. Let's talk a little bit about metric design. One is once you have the metric, but I often find people discount the metrics they have because they know they don't tell the true story.
They see the metric. Then they say, “Oh well. That doesn't really mean anything because the weather was bad,” “There weren't enough days in the month” or whatever the reason is that was a perfectly good explanation to not ask any more questions.
Is that because we just don't know how to design good metrics, or have we not really figured out that we should be designing the metrics? Are we just taking the metrics that naturally come out of the system and measuring them assuming that they're the most useful things?
Mark: That's a good question. There are really good books out there if we're looking in the context of strategy deployment, of asking, “What should we measure? Can we have a balanced scorecard?” There's a book, going back in time, “The Balanced Scorecard.” What measures are not just easy to measure, but what measures indicate the health of our organization or our department?
Those are really, really important questions. My book admittedly doesn't get into that real deeply. There's an element of what I hear in the scenario you posed, Jamie, where there's this defensiveness of when we see a change in the metric, people make excuses. They tell a story. A lot of organizations have this fear associated with a metric.
The metric is changed. People are afraid. It's not hitting the target. People are afraid. One thing I do touch on in the book is when there's fear and when people are put under pressure to hit the target no matter what, people often end up distorting the system or distorting the metric instead of improving the system.
I don't know if that's what you were alluding to. I'd be curious to hear some of your thoughts on that topic.
Jamie: Certainly, I think that's part. Part of it is the reaction itself. Part of it as, if you go back to say Tom Johnson's work of “Profit Beyond Measure,” of how to design a metric that really tells you what's going on. You say, “Hey, our inventory goes up. Well, that's because we're growing significantly. Well then, that's not the right metric. We should be measuring that as a ratio against days of sales.”
The design of the metric, when people start to discount it, I usually start to ask, how do we incorporate those things that are excuses and legitimate excuses into the metric itself without turning it into a convoluted thing that nobody can ever recognize again? There is a balance between simplicity and truthfulness or usefulness.
Mark: There's probably also a balance, I'm sure, as you work with people, on leading indicators, lagging indicators. You could call them process metrics or end result metrics, and finding that right balance, right?
Jamie: Yeah. Obviously, you need to know where those end metrics are taking you. Ultimately, they might cause you to react, but they're not going to tell you how to react. That's where a leading indicator should be more informative.
Mark: If a company, whether it's a big corporation, small business, nonprofit hospital, is measuring just their monthly or quarterly bottom-line number, knowing that profitability is higher and saying, “Well, OK. Is that noise, or is that a signal?”
Either way, that doesn't necessarily point you in the right direction of the different levers you would need to pull or the different improvements you would need to make to either increase revenue or reduce cost. You'd continue breaking down the components of bottom-line profit into things that can be measured more frequently at value stream, business unit or department levels, right?
Jamie: Absolutely. It leads into another question though that's related to that financial look-in on performance and what we measure there. How much of the reaction mentality is dependent, although it may not be driven by, short-term thinking from Wall Street or activist investors knowing it doesn't matter what the trend is this quarter?
People are either going to react or they're not. That's fundamentally outside my control. How does that influence the behaviors that you're seeking through the book?
Mark: That's a good question. A lot of the financial news is really focused on two data point comparisons, whether it's profitability, stock price, the market, economic indicators. Housing starts are down 2.1 percent. That probably doesn't mean the housing market is in a total free fall. It's a data point. Process behavior charts put data into better context, so we can see, is that worth freaking out about?
In some settings, a 2.1 percent change could be a very meaningful number. In some settings, 2.1 percent is just the typical fluctuation. It's down 2.1 percent, but last quarter it was up 2.4 percent. It just maybe fluctuates like that.
Whether it's a financial metric or a safety metric, a quality metric, any other things that we should be measuring in our balanced scorecard, I think we'll do better to understand when a change in the metric is meaningful or not. I'd be curious, you're making me think and wonder, is there an investment strategy around being a contrarian to other people's over-reactions to a single data point?
If a company's profits are down last quarter and lots of people are selling off, and we look at a chart and say “You know, it tends to fluctuate. It's probably going to bounce back next quarter,” is that a buying opportunity? I'm by no means a stock-picker or an investor, but maybe there's opportunity.
Jamie: I do know those strategies exist. That's why we have plenty of statisticians involved in funds today.
Mark: If we believe in the efficient market theory, knowledge of variation would be baked into the collective wisdom of the market, but maybe not.
Jamie: It would. We would hope it eventually would. Speaking of another meta-factor, you mention in the book big data, which of course is just a fancy name for we have more data today than we used to have. It really is still the idea that we've developed the tools to generate lots and lots of data. We probably lack the tools to turn it into information.
The field of data science, which is really a combination of statisticians plus computer scientists, is attempting to change that through AI and other methods. Do you see that trend changing what we're talking about? To make it a very provocative statement, will it kill the simplicity of the process chart because we have AI to figure these things out for us.
Mark: That's a really good question. One of my classmates from my year at MIT, a friend of mine, John Miller, who's done a lot of lean and Six Sigma work in his career, on the last couple years has taken a big interest in a lot of this big data analytics. Maybe I should have him on the podcast sometime to explore some of those topics.
There might be a temptation with big data to capture more metrics more frequently. I see this in hospitals, even if they're not calling it big data or analytics, there's often a good reason in lean management to measure certain metrics on a daily basis in real time instead of monthly indicators that are always a month or two behind.
Generally, I would love to have a daily metric instead of a monthly metric. I would use the methods of process behavior charts to not overreact to those daily fluctuations which are going to tend to be bigger than a monthly fluctuation.
It seems like these rules are pretty timeless. There's probably other opportunities through number crunching to look for correlations and to really take deep dives that maybe help us understand our systems a little bit better. I don't know enough about some of those other topics to say. I don't think it makes these rules for detecting signals in a metric. I don't think it makes it obsolete.
Maybe there's a way of answering questions around the causes of variation in a system that might only come from a really deep, analytical, number crunching of looking for correlations in customer behavior, demographics, and things that might be useful that these charts wouldn't tell you.
I think you said this earlier, and I'll amplify the idea. A process behavior chart will tell you something has changed, it won't tell you what changed. Maybe some of these analytical methods in addition to, I still don't think, it's the idea of going to the Gemba, looking at the process, talking to the people doing the work, that's not obsolete.
I certainly don't want anyone to have an impression that I think metrics are a replacement for all of that. I think the reality is we have metrics. Let's use metrics in a way that better prioritizes our visits to the Gemba, our A3s, our root-cause analysis, and then use it in conjunction with all of these other pretty timeless lean methods.
Jamie: That's a pretty good summary of how it all comes together. It's not a standalone, it's one tool leads to a next, one question leads to another question, and all this has to fit together in the universe in which we operate.
Wrapping things up, you've been teaching this for a while in your talks in your own seminars. You've written about it. You've certainly written about the other big influence, Deming, for a long, long time. You now have a book that brings these ideas closer to the management practice. What are your hopes about the influence? Where does it go from here? What do you hope to change because of this book?
Mark: I was about to say in my wildest dreams. These dreams might not sound that ambitious, but what I would love to see this book has an impact in the hospital space. As you said at the beginning, this is the first book I've written that's not geared only for a healthcare audience. There are examples in the book here from healthcare, startups, and other types of businesses.
When I go and visit a hospital, I'm out in the Gemba, and I see a team's huddle board, I don't want to see the spreadsheet grid of numbers hosted anymore. I'd love to see that if a department has six metrics, instead of a spreadsheet with six line items and some red-green color coding of are we better than our target or not, I'd rather see six charts.
Even if it's the most basic of Excel line charts or some people would call it a run chart, just plot. There's this group in England that's spreading these ideas in the national health service. They use a hashtag #plotthedots. When in doubt, create a visual, a graph instead of a list of numbers. The human brain can see trends or the lack of trends much more clearly if we just create a simple chart.
Better yet would be overlaying the three lines of the average, and the lower limit, and the upper limit. I would hope people would start doing that. I hope people would start saying now that we don't react to every change and every metric equally, we're better prioritizing our improvement effort, and we're actually seeing bigger gains in our performance metrics.
Those are some of the things I would hope to see. As people have gone back and tried to experiment with these methods, that's what I hear reports of.
Instead of giving equal reaction to all six metrics, we focus on the one or the two that tell us something, tell us that it's really worth investigating and focusing on. That hopefully reduces, if you will, some management waste and allows everyone to be more effective in the work they're doing in running and improving their business.
Jamie: That's a pretty observable set of behaviors we can probably all be on the lookout for. I want to wrap things up. This is your stand-in host, Jamie Flinchbaugh, replacing your regular host, Mark Graban, who happens to be our guest today, Mark Graban.
Thanks for talking and sharing the ideas of your book. I'm sure many of your readers and listeners will be picking it up as they usually do, certainly with the last books you've written. I hope it has exactly the influence that you intend it to have.
Mark: I hope so. I appreciate you doing the guest hosting here. I alluded earlier we had done this at least once before. I did take a second to look up here. We did do it once. It was episode 50. We were celebrating the 50th podcast. We're now up over 300. [laughs]
Jamie: That's quite a run then.
Mark: The date of that episode being released was August 7th, 2008 so just barely over 10 years ago if you can believe it.
Jamie: No wonder I couldn't remember exactly. A lot has happened since then. I'm glad to do it again. Maybe we'll have to do this before another 10 years goes by.
Mark: Yeah. I want to thank you. My search here also pulled up some guest posts that you used to do on my blog. I would encourage the listeners go check out Jamie's website, jflinch.com, his blog, his books. You reduced, you went from jamieflinchbaugh.com to jflinch.com. Were those other letters waste?
Jamie: Yeah, the other letters were like…You name your company after yourself, but if you use your full name, it's a little too much, especially with mine.
Mark: Yeah. [laughs] Easier to type and easier to spell, jflinch.com.
For anyone who wants to learn more about my book and see how you can order it, you can go to www.measuresofsuccessbook.com. You can find it in the Amazon Kindle store. You can find it in the Apple iBooks store. That's just about to be renamed just Apple Books. They're eliminating a letter. It will be a paperback book later this year. I hope people will check that out.
I appreciate the indulgence of being interviewed [laughs] and promoting my own book here. Jamie, thank you for doing that. Thank you for asking really good questions.
Jamie: Thank you. Happy reading, everyone.
What do you think? Please scroll down (or click) to post a comment. Or please share the post with your thoughts on LinkedIn – and follow me or connect with me there.
Did you like this post? Make sure you don't miss a post or podcast — Subscribe to get notified about posts via email daily or weekly.
Check out my latest book, The Mistakes That Make Us: Cultivating a Culture of Learning and Innovation: